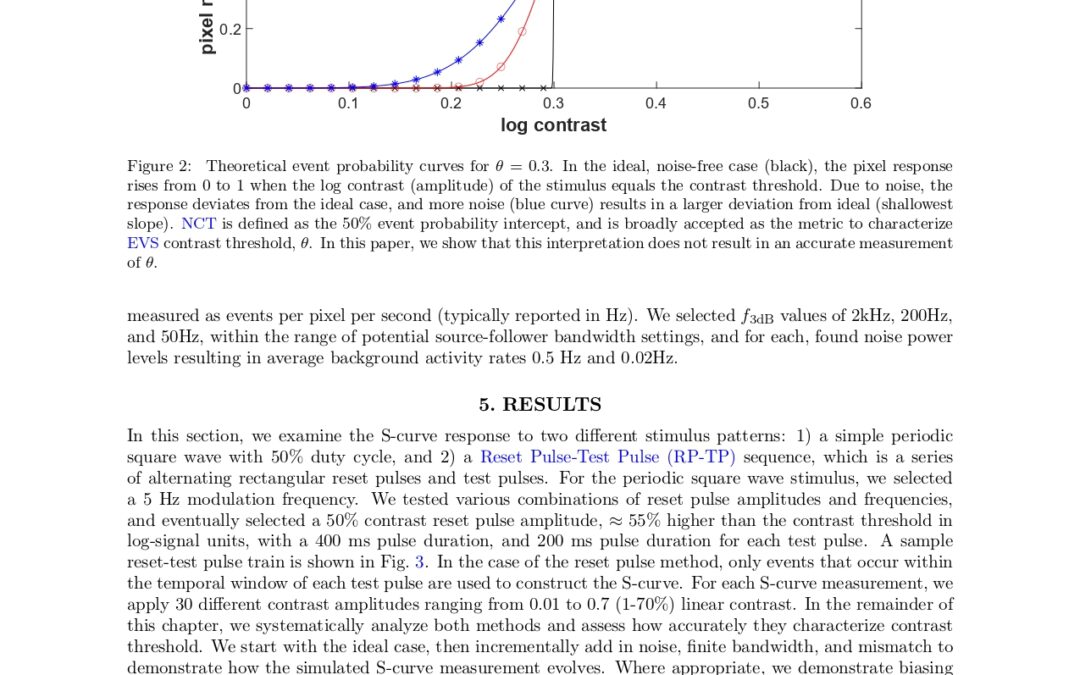
Re-interpreting the Step-Response Probability Curve to Extract Fundamental Physical Parameters of Event-Based Vision Sensors
In this work, we detail the method for generating accurate S-curves by applying an appropriate stimulus and sensor configuration to decouple 2nd-order effects from the parameter being studied. We use an EVS pixel simulation to demonstrate how noise and other physical constraints can lead to error in the measurement, and develop two techniques that are robust enough to obtain accurate estimates.
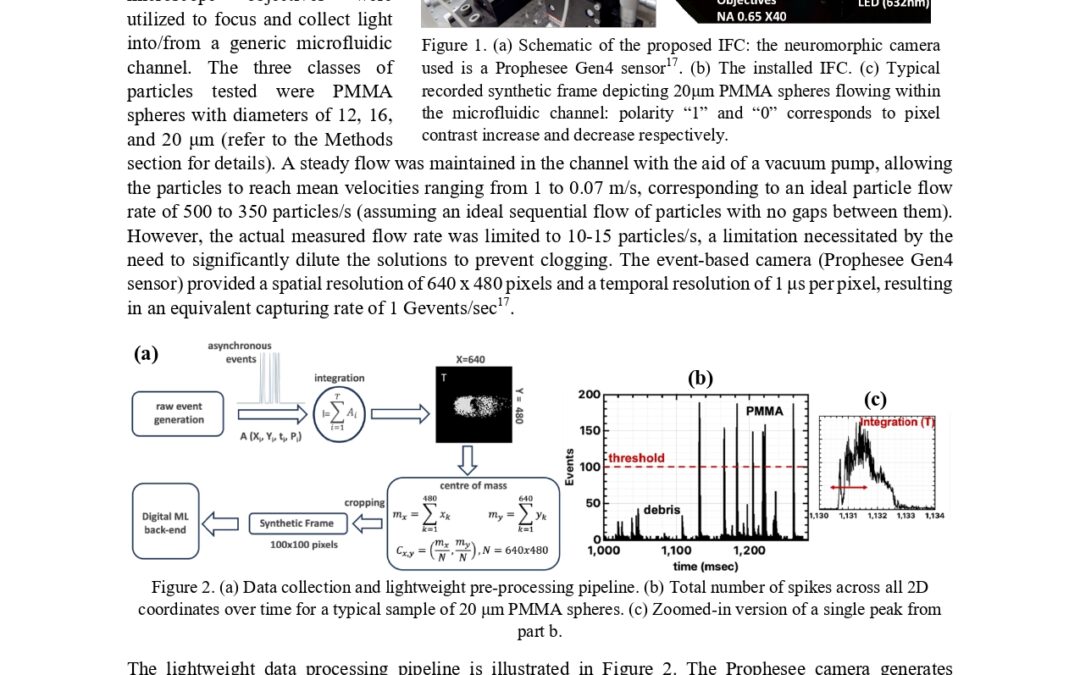
Photonic Neuromorphic Accelerators for Event-Based Imaging Flow Cytometry
In this work, we present experimental results of a high-speed label-free imaging cytometry system that seamlessly merges the high-capturing rate and data sparsity of an event-based CMOS camera with lightweight photonic neuromorphic processing. The results confirm that neuromorphic sensing and neuromorphic computing can be efficiently merged to a unified bio-inspired system, offering a holistic enhancement in emerging bio-imaging applications.
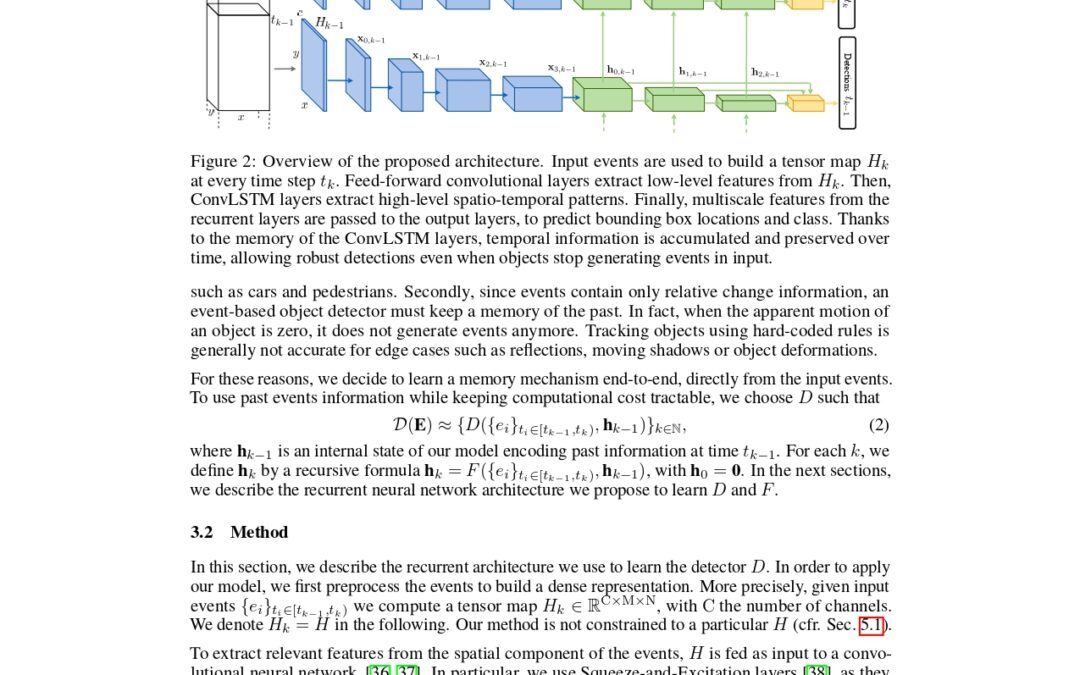
Learning to Detect Objects with a 1 Megapixel Event Camera
Our model outperforms by a large margin feed-forward event-based architectures. Moreover, our method does not require any reconstruction of intensity images from events, showing that training directly from raw events is possible, more efficient, and more accurate than passing through an intermediate intensity image.
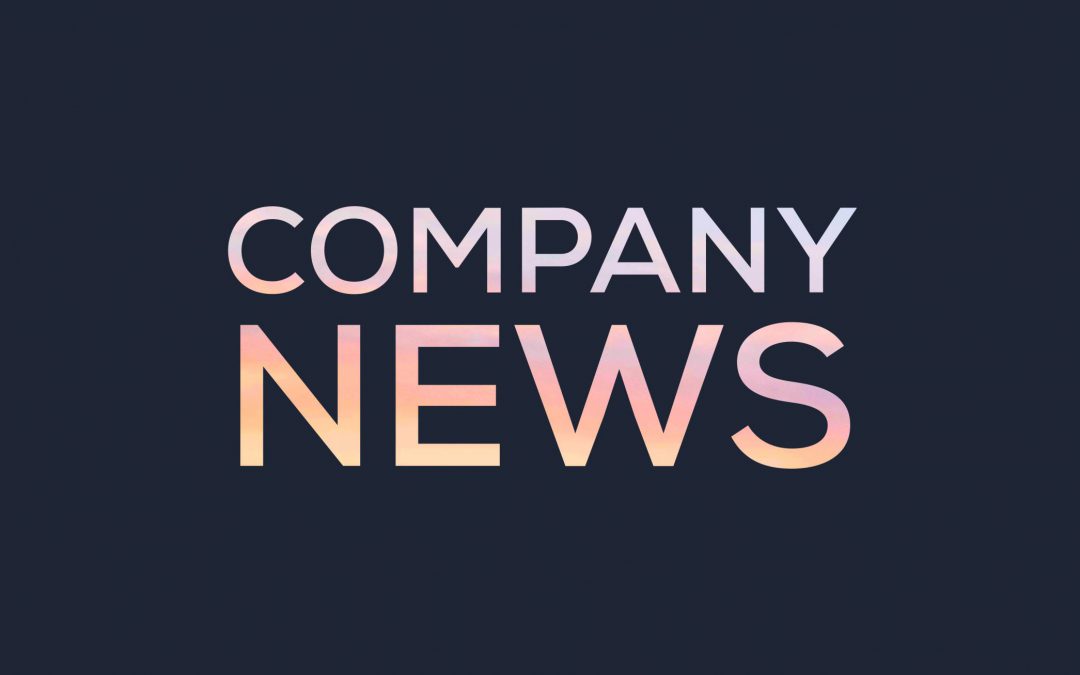
Prophesee announces the appointment of Cyrille Soubeyrat as VP of Software
Prophesee welcomes software R&D expert Cyrille Soubeyrat as new VP of Software